Blog and News
Data Maturity: The Key to Successfully Implementing AI in International Development
By Principal Data Engineer, Ivonne Carrillo
Introduction
The use of data is key for progress and innovation across various sectors, including international development. From monitoring and evaluating the effectiveness of interventions to identifying trends and predicting future needs, data has proven to be an invaluable asset in the quest for sustainable development. However, as the volume and complexity of data continue to grow, the need for advanced tools to process and derive meaningful insights from it becomes increasingly important. This is where artificial intelligence (AI) can help international development organizations (IDOs) approach data and, ultimately, result in better outcomes for the communities they serve.
IDOs have long been using data to inform their decision-making processes. For instance, the World Food Programme (WFP) uses AI and satellite imagery to enable real-time insights and actionable intelligence for effective decision-making during disaster response. Similarly, the United Nations Children's Fund (UNICEF) collects and analyzes data on various indicators, such as child mortality, nutrition, and education, to design and implement targeted interventions.
At Bixal, we have observed that IDOs are struggling with the overwhelming volume and complexity of data. While access to data is not the problem, manual data processing and analysis are time-consuming and often prone to errors.
By automating data processing and analysis, AI can help IDOs in making sense of the vast amounts of data available to them, enabling them to identify patterns, trends, and insights that might otherwise go unnoticed. AI is a tool that can help them unlock the full potential of their data and, in turn, drive more effective and efficient development efforts.
Data maturity refers to how effectively and efficiently an organization manages, analyzes, and uses its data. In a mature data environment, data is well-structured, easily accessible, and consistently reliable for all stakeholders. This foundation of data maturity is crucial for automating data processing and analysis with AI tools because it ensures the readiness and quality of data for efficient and accurate analysis. A higher level of data maturity indicates that an organization has well-structured, clean, and consistent data, which is essential for AI algorithms to operate effectively and generate meaningful insights. Without a sufficient level of data maturity, AI tools may struggle to interpret the data accurately, leading to suboptimal outcomes or erroneous conclusions, thus undermining the potential benefits of automation and advanced analytics in decision-making processes.
Navigating the Risks of AI in International Development: The Importance of Data Maturity
While the potential benefits of AI in international development are undeniable, it is essential to acknowledge and address the risks associated with its implementation. Some of these risks include:
- IDOs handle vast amounts of sensitive data, including personally identifiable information (PII), which must be protected from unauthorized access and use.
- AI algorithms learn from data, and if that data is biased or incomplete, the AI system will reflect and perpetuate that bias. In the context of international development, this could lead to ineffective or even harmful interventions.
- The implementation of AI systems can exacerbate inequalities, particularly if they are not accessible to all communities. It is essential for IDOs to take steps to ensure that AI systems are accessible to all communities, regardless of their socioeconomic status or geographic location.
- AI systems can be vulnerable to cyberattacks, and the compromise of an AI system can have serious consequences, particularly if it involves the handling of sensitive data.
Given these risks, evaluating an organization's data maturity before jumping into AI is crucial. Data maturity acts as a safety net, helping you evaluate your current position and identify desired goals for improvement.
Data Maturity Pyramid: Building a Solid Foundation for AI in International Development
The successful integration of AI is a gradual process, one that begins with a solid foundation and progresses step by step. In this section, we will explore the Data Maturity Pyramid, a strategic framework that will help IDOs scale up their data maturity level, ultimately setting the stage for successful AI efforts.
The Data Maturity Pyramid is a visual representation of the strategic principles that IDOs can follow to build a solid foundation for their AI efforts. Each fundamental concept in the pyramid represents a specific data practice, ultimately helping IDOs reach a mature framework where they have a solid foundation for their informed decision-making and AI initiatives.
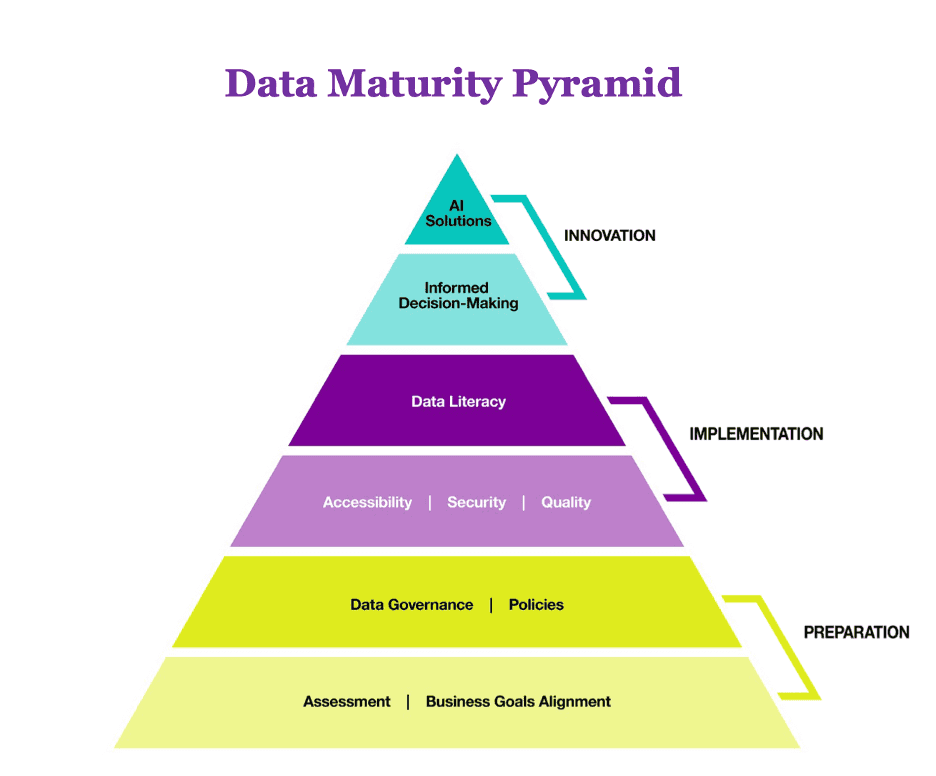
Preparation Strategic Principles
Assessment
To determine where an organization stands in terms of data maturity and to identify the specific steps needed to build a solid foundation for any AI efforts, you need to conduct a self-assessment. The self-assessment process involves evaluating your organization's current data practices from the Data Maturity Pyramid against the five levels of the Data Maturity Model:
- Chaotic or ad hoc: Data is recognized as valuable, but organizations lack a clear data strategy and have limited or no data governance policies in place. Data is collected in an ad hoc manner, and there is little to no consistency in data management and analysis.
- Repeatable: Organizations have established data collection and storage processes. As effective data management practices begin to emerge, they start to see initial levels of standardization.
- Defined: Organizations have a clear data strategy and have defined data governance policies and procedures. Data is collected, managed, and analyzed in a consistent manner, and data quality is a priority.
- Managed: Organizations have implemented tools and technologies to automate data management and analysis processes. Data is closely monitored and measured, and data quality is consistently high.
- Optimized: Data insights drive continuous improvement and innovation, fostering a more evidence-based organization.
Business Goals Alignment
As you progress to business goals alignment, it is important to regularly review and iterate upon your roadmap to ensure that it remains in line with your organization's evolving objectives.
Additionally, all stakeholders in the data maturity assessment and AI implementation process must be involved to ensure that everyone is on the same page and that the AI solutions are being implemented in a way that supports an organization's strategic objectives.
Data Governance and Policies
Strong data governance policies ensure data accuracy, security, and compliance. IDOs must evaluate their data protection policies and procedures to ensure that they are robust enough to address the challenges posed by AI. These policies inform decisions on data access, retention, and ownership, which are critical when working with sensitive data.
Implementation Strategic Principles
Data Accessibility
Restricted data access can hinder the development and deployment of AI solutions. Data silos resulting from inadequate access to critical data across teams and departments can limit the potential for collaboration. To overcome these barriers, IDOs must adopt a collaborative approach to data sharing, and data accessibility must be balanced with data privacy concerns and robust security measures., i.e., organizations must ensure that sensitive data is protected while still allowing for appropriate access to data. This may involve implementing role-based access control, data masking, or other access control mechanisms. The timely delivery of relevant data to the right people should be incorporated into data policies.
Data Quality
Poor quality data can significantly impact the accuracy and reliability of AI models. In the context of international development, biased or incomplete data could lead to ineffective or even harmful interventions. For example, an AI system trained on biased data may wrongly determine certain groups to be more vulnerable to a specific challenge, disregarding the actual groups that may be more at risk. It is essential for organizations to establish tools and metrics to measure data quality and representativeness of their data before implementing AI systems, and to continuously monitor and address any biases that may arise.
Data Security
IDOs should evaluate their security policies and procedures to ensure that they are robust enough to address the challenges posed by AI, including data protection, encryption, and data privacy. By implementing appropriate security measures, organizations can protect against data breaches, unauthorized access, and other security threats, and build trust with the communities they serve.
Data Literacy
Cultivating data literacy within your organization is essential for optimizing AI implementations and facilitating faster adoption of cutting-edge technologies.
People with different roles within an organization, from data scientists to program managers, must cultivate a shared understanding of data practices to ensure efficient problem-solving and effective communication when discussing data storage, accessing information, describing data, and extracting insights from datasets.
Data literacy also encourages collaboration and knowledge sharing, as team members work together to analyze and interpret data, and make informed decisions based on the insights gained. Additionally, an organization will be better equipped to identify opportunities to work with AI tools and technologies.
To cultivate data literacy within your organization, implement training programs, workshops, and other learning opportunities. Also, consider establishing a data literacy framework that outlines the key concepts, skills, and tools required for working with data.
Innovation: The Ultimate Goal
As organizations aim to mature their data practices, the ultimate goal is to drive innovation
Informed Decision-Making
By using data analysis tools that are easily accessible to everyone within the organization, a foundation for good decision-making is set. Regular insights and reports should be provided to stakeholders to ensure that data isn't just collected but is actively used to guide the decision-making process. This approach ensures that every decision is backed by data, optimizing outcomes and steering strategies in the right direction
Moreover, the impact of these informed decisions isn't left to guesswork. It's measured through clear metrics such as reach (the population served), revenue, cost, and customer satisfaction
AI Solutions
Implementing AI solutions is the culmination of the data maturity journey. Organizations must identify the right applications for AI and machine learning and ensure that the data is suitably prepared for these advanced models. Deploying AI and machine learning tools and platforms with a focus on monitoring and evaluating their performance and accuracy—through metrics such as precision, recall, and F1 score—signifies an organization's commitment to leveraging cutting-edge technology for insight-driven decision-making
Conclusion
The integration of AI in international development holds immense potential for driving more effective and efficient development efforts—from enhancing healthcare outcomes and optimizing resource allocation to providing predictive interventions and personalized support.
However, it is essential to remember that the successful implementation of AI is a gradual process that begins with a foundation. By following the Data Maturity Pyramid, IDOs can harness the full potential of mature data to power AI solutions that improve lives globally. And by embracing an evidence-driven culture, IDOs can adapt to changing circumstances, meet the needs of the most vulnerable, and contribute to a more equitable and prosperous world.
For a comprehensive guide on leveraging your organization's data potential to drive innovation, be sure to explore our printable Data Maturity Checklist.